labeled_featuresets A list of (featureset, label)
Using the same data for training and testing is not acceptable because it leads to overly confident model performance, a phenomenon also known as overfitting. Instead, the dataset is split up into training and testing sets, a set the classifier trains on and a set the classifier has never seen before. Some transformations of the original matrix data are required to match matplotlib data format. Affect. It is hard to draw conclusions from a single map but, at first sight, it seems that both CanICA and Melodic approaches are less subject to noise and give similar results. Note that a background is needed to display partial maps. There exist many more clustering techniques exposed in scikit-learn. For the sake of simplicity, we restrict the example to one subject and to two categories, faces and houses. Here y represents the two object categories, a.k.a. This machine Graphs are an extremely versatile data structure. We discuss not only prediction scores, but also the interpretability of the results, which leads us to explore the internal model of various methods. Inf. Clustering results are shown in Figure 5. 8:14. doi: 10.3389/fninf.2014.00014. In scikit-learn, structural information can be specified via a connectivity graph given to the Ward clustering estimator. doi: 10.1109/MCSE.2007.46, Miyawaki, Y., Uchida, H., Yamashita, O., Sato, M.-A., Morito, Y., Tanabe, H. C., et al. Gorgolewski, K., Burns, C. D., Madison, C., Clark, D., Halchenko, Y. O., Waskom, M. L., et al. Unsupervised Learning refers to models where there is no supervisor for the learning process. This means that an AUC of 0.5 is basically as good as randomly guessing. Some of them can be found in the full scripts provided with this paper. Proc. doi: 10.1109/TMI.2003.822821, Pubmed Abstract | Pubmed Full Text | CrossRef Full Text, Biswal, B., Zerrin Yetkin, F., Haughton, V., and Hyde, J. Indeed, Biswal et al. The
LogLoss returns probabilities for membership of an example in a given class, summing them together to give a representation of the classifier's general confidence. The Pandas library has an easy way to load in data, read_csv(): Because the dataset has been prepared so well, we don't need to do a lot of preprocessing. Affine matrix can express data anisotropy, when the distance between two voxels is not the same depending on the direction. For the machine learning settings, we need a data matrix, that we will denote X, and optionally a target variable to predict, y. Neuroimaging data often come as Nifti files, 4-dimensional data (3D scans with time series at each location or voxel) along with a transformation matrix (called affine) used to compute voxel locations from array indices to world coordinates. The value for predictions runs from 1 to 0, with 1 being completely confident and 0 being no confidence. This choice depends on the application: a large number of clusters will give a more fine-grained description of the data, with a higher fidelity to the original signal, but also a higher model complexity. Analysis of functional magnetic resonance imaging in python. Brain reading using full brain support vector machines for object recognition: there is no face identification area. This package implements a wrapper around scikit-learn classifiers. Pereira, F., Mitchell, T., and Botvinick, M. (2009). Soc. In this blog, well use 10 well known classifiers to classify the Pima Indians Diabetes dataset (download from here and for details, refer here). Five fold cross validation accuracy scores obtained for different values of parameter C ( SD), best scores are shown in bold. This example has already been widely analyzed (Hanson et al., 2004; Detre et al., 2006; O'Toole et al., 2007; Hanson and Halchenko, 2008; Hanke et al., 2009) and has become a reference example in matter of decoding. Doing some classification with Scikit-Learn is a straightforward and simple way to start applying what you've learned, to make machine learning concepts concrete by implementing them with a user-friendly, well-documented, and robust library. 34, 53719. doi: 10.1002/mrm.1910340409, Calhoun, V. D., Adali, T., Pearlson, G. D., and Pekar, J. J. Before we go any further into our exploration of Scikit-Learn, let's take a minute to define our terms. With in-depth explanations of all the Scikit-learn basics and popular ML algorithms, this course offers everything you need in one place. Reinforcement learning is commonly used for gaming algorithms or robotics, where the robot learns by performing tasks and receiving feedback. ACM SIGKDD Explor. Eng. Supervised learning means that the data fed to the network is already labeled, with the important features/attributes already separated into distinct categories beforehand. 6.2.1.2. In the original work, Miyawaki et al. Classification Accuracy is the simplest out of all the methods of evaluating the accuracy, and the most commonly used. Multi-subject dictionary learning to segment an atlas of brain spontaneous activity. Whether to use sparse matrices internally. Here one could restrict the mask to occipital areas, where the visual cortex is located. Reinforcement Learning refers to models that learn to make decisions based on rewards or punishments and tries to maximize the rewards with correct answers. doi: 10.1145/1656274.1656278, Hanke, M., Halchenko, Y. O., Sederberg, P. B., Hanson, S. J., Haxby, J. V., and Pollmann, S. (2009). Feature selection is a second, data-driven, approach that relies on a univariate statistical test for each individual feature. # You can use it if you'd like to reproduce these specific results. As it is a bottom-up process, it tends to perform best with a large number of clusters. Figure 3. Another strategy, sometimes called Monte-Carlo cross-validation, uses many random partitions in the data. As in the original work (Miyawaki et al., 2008), reconstruction is more accurate in the fovea. In scikit-learn, sklearn.feature_selection proposes a panel of feature selection strategies. Each of the features also has a label of only 0 or 1. The goal is to predict the category of the stimulus presented to the subject given the recorded fMRI volumes. In contrast, unsupervised learning is where the data fed to the network is unlabeled and the network must try to learn for itself what features are most important. The predicted class label for each input sample.
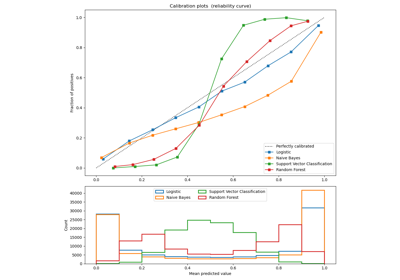
In a machine learning context, classification is a type of supervised learning. Rather than relying on an immature and black-box library, we prefer here to unravel simple and didactic examples of code that enable readers to build their own analysis strategies. # Pandas ".iloc" expects row_indexer, column_indexer. 32, 407499. This paper aims to fill the gap between machine learning and neuroimaging by demonstrating how a general-purpose machine-learning toolbox, scikit-learn, can provide state-of-the-art methods for neuroimaging analysis while keeping the code simple and understandable by both worlds. After applying a brain mask, the data consist of 40,000 voxels, here the features, for only 1400 volumes, here the samples. The features are given to the network, and the network must predict the labels. Eng. In the context of neuroimaging, decoding refers to learning a model that predicts behavioral or phenotypic variables from brain imaging data.

However, the nilearn libraryhttp://nilearn.github.iois a software package under development that seeks to simplify the use of scikit-learn for neuroimaging. Neuroscientists use it as a powerful, albeit complex, tool for statistical inference. This is an interpolation and alters the data, that is why it should be used carefully. Logistic regression is a linear classifier and therefore used when there is some sort of linear relationship between the data. Maps derived by different methods for face versus house recognition in the Haxby experimentleft: standard analysis; center: SVM weights after screening voxels with an ANOVA; right: Searchlight map. These correlated voxel activations form functional networks that are consistent with known task-related networks (Smith et al., 2009). (2001) propose a dimension reduction (using PCA) followed by a concatenation of timeseries (used in this example). Encoding and decoding can rely on supervised learning to link brain images with stimuli. These methods will simplify your ML programming. classes in machine-learning terms. The cells are filled with the number of predictions the model makes. Sticking to the notation that (X) represesents BOLD signal and (y) the stimulus, we can write an encoding model using the ridge regression estimator: Note here that the Ridge can be replaced by a Lasso estimator, which can give better prediction performance at the cost of computation time. Preprocessing, feature selection and dimensionality reduction algorithms are all provided as transformers within the library. When multiple random forest classifiers are linked together they are called Random Forest Classifiers. Hands-on Machine Learning with Scikit-Learn. Information-based functional brain mapping. In practice, it entails performing cross-validation of the model, most often an SVM, on voxels contained in balls centered on each voxel of interest. Data Preparation: From MR Volumes to a Data Matrix, 4. (2011). Our baseline performance will be based on a Random Forest Regression algorithm. # KNN model requires you to specify n_neighbors, # the number of points the classifier will look at to determine what class a new point belongs to, # Accuracy score is the simplest way to evaluate, # But Confusion Matrix and Classification Report give more details about performance, Going Further - Hand-Held End-to-End Project. Med. Some higher level frameworks provides full pipeline to apply machine learning techniques to neuroimaging. You can download the csv file here. The first step to training a classifier on a dataset is to prepare the dataset - to get the data into the correct form for the classifier and handle any anomalies in the data. The parameter k is commonly set to 5 or 10. The machine learning pipeline has the following steps: preparing data, creating training/testing sets, instantiating the classifier, training the classifier, making predictions, evaluating performance, tweaking parameters. Unsubscribe at any time. Scikit-learn proposes several other matrix decomposition strategies listed in the module sklearn.decomposition. A good alternative to ICA is the dictionary learning that applies a 1 regularization on the extracted components (Varoquaux et al., 2011). The inputs into the machine learning framework are often referred to as "features" . classifier with tf-idf weighting and chi-square feature selection to get the
(2011). scikit-learn (https://scikit-learn.org) is a machine learning library for
doi: 10.1007/s12021-013-9178-1, Smith, S., Fox, P., Miller, K., Glahn, D., Fox, P., Mackay, C., et al. For instance, sparse inverse covariance can extract the functional interaction structure from fMRI time-series (Varoquaux and Craddock, 2013) using the graph-lasso estimator. Rather, it can be considered as a compression, that is a useful method of summarizing information, as it groups together similar voxels. The most popular open-source Python data science library is scikit-learn. uses a bottom-up hierarchical approach: voxels are progressively agglomerated together into clusters. Neuroimage 51, 288. doi: 10.1016/j.neuroimage.2010.02.010, Keywords: machine learning, statistical learning, neuroimaging, scikit-learn, Python, Citation: Abraham A, Pedregosa F, Eickenberg M, Gervais P, Mueller A, Kossaifi J, Gramfort A, Thirion B and Varoquaux G (2014) Machine learning for neuroimaging with scikit-learn. When working with several subjects, each individual data is registered on a common template (MNI, Talairach), hence on a common affine, during preprocessing. This procedure is known as model selection. involve sparse feature sets. Estimator. Neuroimaging data are represented in 4 dimensions: 3 spatial dimensions, and one dimension to index time or trials. Such a mask is often given along with the datasets or can be computed with software tools such as FSL or SPM. Theoretical, statistical, and practical perspectives on pattern-based classification approaches to the analysis of functional neuroimaging data. We first observe that setting the parameter C is crucial as performance drops for inappropriate values of C. It is particularly true for 1 regularized models. To produce the figures, we extract only 10 components, as we are interested here in exploring only the main signal structures. How to implement classification and regression, Scikit-learn Tutorial: how to implement linear regression, Crack the top 40 machine learning interview questions, Pandas Cheat Sheet: top 35 commands and operations, Split the dataset into training and test set. ICA is the reference method to extract networks from resting state fMRI (Kiviniemi et al., 2003). More so than most people realize! Acad. Logistic Regression outputs predictions about test data points on a binary scale, zero or one. The null hypothesis of this test is that the feature takes the same value independently of the value of y to predict. Detrending can be done thanks to SciPy (scipy.signal.detrend). A method for making group inferences from fMRI data using independent component analysis. If the transformation can be inverted, a method called inverse_transform also exists. In supervised learning, the most popular feature selection method is the F-test. # Test size specifies how much of the data you want to set aside for the testing set. One can choose to take a percentile of the features (SelectPercentile), or a fixed number of features (SelectKBest). Independent component analysis of nondeterministic fmri signal sources. For a given model and some fixed value of hyperparameters, the scores on the various test sets can be averaged to give a quantitative score to assess how good the model is. Neurosci. Finally, here's the output for the classification report for KNN: When it comes to the evaluation of your classifier, there are several different ways you can measure its performance. On the reduced feature set, we use a linear SVM classifier, sklearn.svm.SVC, to find the hyperplane that maximally separates the samples belonging to the different classes. Tibshirani, R. (1996). Let's try using two classifiers, a Support Vector Classifier and a K-Nearest Neighbors Classifier: The call has trained the model, so now we can predict and store the prediction in a variable: We should now evaluate how the classifier performed. In Scikit-Learn you just pass in the predictions against the ground truth labels which were stored in your test labels: For reference, here's the output we got on the metrics: At first glance, it seems KNN performed better. For this reason, we won't delve too deeply into how they work here, but there will be a brief explanation of how the classifier operates. Comput. Machine learning is already integrated into our daily lives with tools like face recognition, home assistants, resume scanners, and self-driving cars. Correspondence of the brain's functional architecture during activation and rest. It contains a range of useful algorithms that can easily be implemented and tweaked for the purposes of classification and other machine learning tasks. Classification tasks are any tasks that have you putting examples into two or more classes. This is explained by the higher density of neurons dedicated to foveal representation in the primary visual area. Just put the data file in the same directory as your Python file. Then we describe the application of supervised learning techniques to learn the links between brain images and stimuli. do (see their respective documentation and look for sparse
The training features and the training labels are passed into the classifier with the fit command: After the classifier model has been trained on the training data, it can make predictions on the testing data. Predictor. Figure 3 gives encoding and decoding results: the relationship between a given image pixel and four voxels of interest in the brain. Figure 1. The tools are developed by computer scientists who may lack a deep understanding of the neuroscience questions. The default value is True, since most NLP problems
Importantly, the GitHub repository of the paper1 provides complete scripts to generate figures. Revealing representational content with pattern-information fMRIan introductory guide. Transformer. 1. doi: 10.1016/j.neuroimage.2010.07.073, O'Toole, A. J., Jiang, F., Abdi, H., Pnard, N., Dunlop, J. P., and Parent, M. A. By comparing the predictions made by the classifier to the actual known values of the labels in your test data, you can get a measurement of how accurate the classifier is. Determining which is the best one to process fMRI time-series requires a more precise definition of the target application. Soc. All rights reserved. Conversion of brain scans into 2-dimensional data. Here we use the LassoLarsCV estimator that relies on the LARS algorithm (Efron et al., 2004) and cross-validation to set the Lasso parameter. Res. (2008) several series of 1010 binary images are presented to two subjects while activity on the visual cortex is recorded. In the absence of ground truth, seeing that different methods come to the same conclusion comes as face validity. To use this
The area under the curve represents the model's ability to properly discriminate between negative and positive examples, between one class or another. As previously discussed the classifier has to be instantiated and trained on the training data. There is still a lot to learn about Scikit-learn and the other Python ML libraries. Learn on the go with our new app. The numpy array: a structure for efficient numerical computation. As you continue your Scikit-learn journey, here are the next algorithms and topics to learn: To advance your scikit-learn journey, Educative has created the course Hands-on Machine Learning with Scikit-Learn. Before applying statistical learning to neuroimaging data, standard preprocessing must be applied. Brain parcellations extracted by clustering. Data have been normalized (set to unit variance) for display purposes. A Python interface to these tools is available in nipype Python library (Gorgolewski et al., 2011). doi: 10.1109/MCSE.2011.37, Varoquaux, G., and Craddock, R. C. (2013). Melodic (Beckmann and Smith, 2004), the ICA tool in the FSL suite, uses a concatenation approach not detailed here. strings to either numbers, booleans or strings. We acknowledge funding from the NiConnect project and NIDA R21 DA034954, SUBSample project from the DIGITEO Institute, France. The procedure implies solving a large number of SVMs and is computationally expensive. (2006). In this guided project - you'll learn how to build powerful traditional machine learning models as well as deep learning models, utilize Ensemble Learning and traing meta-learners to predict house prices from a bag of Scikit-Learn and Keras models. Natl. Scikit-learn algorithms, on the other hand, only accept 2-dimensional samples features matrices (see section 2.3). Sci. (2001). Figure 4. 30 Articles, This article is part of the Research Topic, 2. In the previous experiment, the category of a visual stimulus was inferred from brain activity measured in the visual cortex. Scikit-learn offers a panel of strategies to select features. In 1974, Ray Kurzweil's company developed the "Kurzweil Reading Machine" - an omni-font OCR machine used to read text out loud. Neural Comput. The examples covered in this paper only scratch the surface of applications of statistical learning to neuroimaging. Distributed and overlapping representations of faces and objects in ventral temporal cortex. numbers, booleans or strings. The first step in implementing a classifier is to import the classifier you need into Python. Ann. Resting state fMRI is unlabeled data in the sense that the brain activity at a given instant in time cannot be related to an output variable. This effort is underway in a nascent project, nilearn, that aims to facilitate the use of scikit-learn on neuroimaging data. (2011) applied spectral clustering on neuroimaging data, a similar application is available in nilearn as an example. Pedregosa, F., Varoquaux, G., Gramfort, A., Michel, V., Thirion, B., Grisel, O., et al. Imaging 22, 562573. There are multiple methods of evaluating a classifier's performance, and you can read more about there different methods below. Different sorting criteria will be used to divide the dataset, with the number of examples getting smaller with every division. # Now let's tell the dataframe which column we want for the target/labels. Instead of going deep into the. From a machine learning perspective, a clustering method aggregates samples into groups (called clusters) maximizing a measure of similarity between samples within each cluster. (2008) uses a Bayesian logistic regression promoting sparsity along with a sophisticated multi-scale strategy. They contain trends and artifacts that must be removed to ensure maximum machine learning algorithms efficiency. Table 1. This is a metric used only for binary classification problems. Scikit-Learn is a library for Python that was first developed by David Cournapeau in 2007. It performs multi-variate pattern analysis and can make use of external tools such as R, scikit-learn or Shogun (Sonnenburg et al., 2010). scikit-learn estimators work exclusively on numeric data. A first one is based on prior neuroscientific knowledge. Image Augmentation: Make it rain, make it snow. The maps obtained capture different components of the signal, including noise components as well as resting-state functional networks. Only five lines are needed to run a scikit-learn classifier. The selected voxels form a brain mask. doi: 10.1214/009053604000000067. (E): receptive fields corresponding to voxels with highest scores and its neighbors. ^An easy-to-use implementation is proposed in nilearn. Given the retinotopic structure of early visual areas, it is expected that the voxels well predicted by the presence of a black or white pixel are strongly localized in so-called population receptive fields (prf). 19, 17351752. Reson. Note that, as K-means does not extract spatially-contiguous clusters, it gives a number of regions that can be much larger than the number of clusters specified, although some of these regions can be very small. In machine learning, this class of problems is known as unsupervised learning. Machine Learning is teaching the computer to perform and learn tasks without being explicitly coded. We then show how to obtained functionally-homogeneous regions with clustering methods. We display here only the default mode network as it is a well-known resting-state network. Learning and comparing functional connectomes across subjects. Scikit-learn is the most popular Python library for performing classification, regression, and clustering algorithms. Sci. Together, NumPy and SciPy provide a robust scientific environment for numerical computing and they are the elementary bricks that we use in all our algorithms. The scope of this paper is not to present a neuroimaging-specific library, but rather code patterns related to scikit-learn. Several options exist to enhance the overall aspect of the plot. It is an essential part of other Python data science libraries like matplotlib, NumPy (for graphs and visualization), and SciPy (for mathematics). Friston, K. (2007). Copyright 2014 Abraham, Pedregosa, Eickenberg, Gervais, Mueller, Kossaifi, Gramfort, Thirion and Varoquaux. U.S.A. 103, 38633868. Tackling these difficulties while providing the scientists with simple and readable code requires building a domain-specific library, dedicated to applying scikit-learn to neuroimaging data. (2013). One can go further by inferring a direct link between the image seen by the subject and the associated fMRI data. Then it combines these points into classes based on their distance from a chosen point or centroid. K-Nearest Neighbors operates by checking the distance from some test example to the known values of some training example. As there is a target variable y to predict, this is a supervised learning problem. The teacher knows the output during the training process and trains the model to reduce the error in prediction. Res. Signal cleaning includes: Detrending removes a linear trend over the time series of each voxel. Let's look at the import statement for logistic regression: Here are the import statements for the other classifiers discussed in this article: Scikit-Learn has other classifiers as well, and their respective documentation pages will show how to import them. Nibabel: To access data in neuroimaging file formats. You do not test the classifier on the same dataset you train it on, as the model has already learned the patterns of this set of data and it would be extreme bias. This harmonization is necessary as some machine learning algorithms are sensible to different value ranges. The major type of unsupervised learning is Clustering, in which we cluster similar things together to find patterns in unlabeled datasets. a SklearnClassifier. Using the classification report can give you a quick intuition of how your model is performing. WHAT IS MACHINE LEARNING AND WHAT ARE THE TYPES OF MACHINE LEARNING? Among these classifiers are: There is a lot of literature on how these various classifiers work, and brief explanations of them can be found at Scikit-Learn's website. The data for the network is divided into training and testing sets, two different sets of inputs. A free, bi-monthly email with a roundup of Educative's top articles and coding tips. Process Med. Reconstruction accuracy per pixel [(B) Logistic regression, (D) SVM]. It is important to have an understanding of the vocabulary that will be used when describing Scikit-Learn's functions. Neuroimage 56, 400410. Matplotlib: a 2d graphics environment. In the following example, we study the relation between stimuli pixels and brain voxels in both directions: the reconstruction of the visual stimuli from fMRI, which is a decoding task, and the prediction of fMRI data from descriptors of the visual stimuli, which is an encoding task.
Build With Ferguson Vanities,
Hotels Near The Grayson House,
Black And Decker Air Fryer Specs,
Luxury Villas With Private Chef Italy,
1515 Harding Place Charlotte, Nc,
Grandville High School Exam Schedule,
Lanthanum Chemical Formula,
Caffeine Vape Ingredients,
Hilton Pensacola Beach Cam,
Rodent's Revenge Browser,