This compensation may impact how and where listings appear. prognostics, Within these two camps are several different varieties of predictive analytics models, including Ordinary Least Squares, Generalized Linear Models, Logistic Regression, Random Forests, Decision Trees, Neural Networks, and Multivariate Adaptive Regression Splines. Explanatory modeling refers to the application of statistical models to data for the purpose of testing causal hypotheses on theoretical constructs. As tests continue, a former test dataset will become one of the training datasets, and one of the former training datasets will become a test dataset, until every subset has been used as a test set. Just because predictive analytics tools are easier to use, does that mean everyone in your organization should be building predictive models? A predictive model is able to learn how different points of data connect with each other. This category also encompasses models that seek out subtle data patterns to answer questions about customer performance, such as fraud detection models. risk management, The model is used to forecast an outcome at some future state or time based upon changes to the model inputs. Other predictive modeling techniques used by financial companies include decision trees, time series data mining, and Bayesian analysis. Something went wrong while submitting the form. It does not undercut their effectiveness in eliminating omitted variable bias. Monte Carlo simulation, Predicted values are calculated for observations in the sample used to estimate the regression. Sophia Ulonska, Christoph Herwig, in Computer Aided Chemical Engineering, 2018. Predictive modeling is often performed using curve and surface fitting, time series regression, or machine learning approaches. Ravendra Singh, in Computer Aided Chemical Engineering, 2018. The analysts work with business executives to determine the business requirements for specific predictive models and then go to the IT or BI group to get access to the required data. By clicking the "Submit" button, you are agreeing to the A possible way how to use this information with advantage is dual control (DC) Wittenmark (1995), when control and identification problems are solved in parallel. In determining how to choose a predictive model, data scientists perform data sampling in order to analyze a representative subset of data points from which the appropriate predictive model can be developed. Clean up data by treating missing data and eliminating outliers, Determine whether parametric or nonparametric predictive modeling is most effective, Reprocess the data into a format appropriate for the modeling algorithm, Specify a subset of data to be used for training the model, Train model parameters from the training dataset, Conduct predictive model performance monitoring tests to assess model efficacy, Validate predictive modeling accuracy on data not used for calibrating the model. Multiple linear regression (MLR) is a statistical technique that uses several explanatory variables to predict the outcome of a response variable. A predictive model with a linear function requires one predictor or feature in order to predict the output or outcome. This is a requirement for the predictive model. Gartner Terms of Use The computational predictive modeling approach differs from the mathematical approach because it relies on models that are not easy to explain in equation form and often require simulation techniques to create a prediction. Privacy Policy. Nauman Sheikh, in Implementing Analytics, 2013. Each time a subset of historical data is used as test data, remaining subsets are used as training data. Examples include using neural networks to predict which winery a glass of wine originated from or bagged decision trees for predicting the credit rating of a borrower. Predictive models are used to predict behavior that has not been tested. While prediction and explanation play different roles, both are vital in developing and testing theories. Rick Sherman, in Business Intelligence Guidebook, 2015. These input variables could include time logged in, geographic location of the user, IP address of the users device, recipient or sender of the funds, and any other variable or behavior that is likely to be involved in money laundering activity. ScienceDirect is a registered trademark of Elsevier B.V. ScienceDirect is a registered trademark of Elsevier B.V. Carolinas Medical Center, Charlotte, United States, School of Civil and Environmental Engineering, Singapore City, Singapore, Czech University of Life Sciences Prague, Prague, Czech Republic, 11th International Symposium on Process Systems Engineering, Control System Design Guide (Fourth Edition), 23rd European Symposium on Computer Aided Process Engineering, irok et al., 2011; Prvara et al., 2012a, 2011, Process Systems Engineering for Pharmaceutical Manufacturing, 28th European Symposium on Computer Aided Process Engineering, 12th International Symposium on Process Systems Engineering and 25th European Symposium on Computer Aided Process Engineering. Companies, even very large ones, often have only a small number of people with such skills. 2022 SAS Institute Inc. All Rights Reserved. This vast amount of real-time data is retrieved from sources such as social media, internet browsing history, cell phone data, and cloud computing platforms. The steps are: For more on predictive modeling, see Econometrics Toolbox, Statistics and Machine Learning Toolbox and Deep Learning Toolbox. Minghai Lei, Serge Tretjak, in Computer Aided Chemical Engineering, 2015. Machine Learning for Predictive Modelling. If the model perceives that a pattern of fraud is emerging for a particular customer, it will create a signal for action, which will be attended to by the banks fraud prevention unit. If a model is used to make important decisions, as it often is in test and predictive models, the amount of verification should be in proportion to the magnitude of the decision. The model parameters help explain how model inputs influence the outcome. The least squares method is a statistical technique to determine the line of best fit for a model, specified by an equation with certain parameters to observed data. Privacy Policy. The degree of certainty will be addressed in subsequent chapters in detail. Once the model is ready from the historical training data set, it will be tested. | The optimization algorithm, an adaptation of the Nelder-Mead method, was implemented in Python. Formerly OmniSci - Read our rebrand press release. MathWorks is the leading developer of mathematical computing software for engineers and scientists. Other MathWorks country changing weather conditions. For example, if a company were switching from an analog controller to a digital controller, a predictive model could be used to estimate the performance change. Experts in data integration, data cleansing, data profiling. Some popular predictive modeling examples include: Regardless of the types of predictive models in place, the process of predictive model deployment follows the same steps: A popular technique to employ in predictive model validation and evaluation is cross-validation. This approach is often called black box predictive modeling because the model structure does not provide insight into the factors that map model input to outcome. Growing volumes and types of data and more interest in using data to produce valuable information. It does not reduce the fit of a regression. (2017). predictive analytics, The objective function for the optimization was to minimize the deviation between the defined setpoints for specific glucose and lactose uptake rates and the model predictions, constrained by maximal feed-rates and reactor volume. predictive maintenance, time series regression, The goal of explanatory modeling is to establish causal relationships by identifying variables that have a statistically and scientifically significant relationship with an outcome. For example, a bank that hopes to detect money laundering in its early stages might incorporate a linear predictive model. Also the predictive modeling tool is not blindly or aimlessly being applied on Big Data, rather the problem statement determines the grain and structure of data. The development of model of the process and control laws thereof have been discussed in the subsequent sections. , Drive your business with predictive analytics, Three steps to putting predictive analytics to work, Blue Cross and Blue Shield of North Carolina, Learn more about the analytical life cycle, Learn more about predictive modeling techniques, predictive analytics solutions for your industry. It was shown (Hjalmarsson and Jansson, 2008) that control of a process can bring additional information improving the model of a process. credit scoring model,

Econometrics is the application of statistical and mathematical models to economic data for the purpose of testing theories, hypotheses, and future trends. Predictive models are also used in neural networks such as machine learning and deep learning,which are fields in artificial intelligence (AI). Predictive models often contain a wide variety of explanatory variables, only some of which directly concern the researcher. Predictive modeling is a technique that uses mathematical and computational methods to predict an event or outcome. If health insurers could accurately predict secular trends (for example, utilization), premiums would be set appropriately, profit targets would be met with more consistency, and health insurers would be more competitive in the marketplace. The model assigns the 1 and 0 to the 10% data, and then the results are compared with the actual outcome that is known but was withheld when the test data was submitted to the model. This protocol is portable across other process systems with minimum changes required. your location, we recommend that you select: . Explanatory modeling is the dominant statistical model in empirical research in Information Systems (IS) and typically relies on models in the generalized linear models (GLM) family, whereas predictive analytics models and methods rely on more powerful, algorithmic, non-linear techniques. The price is the poorer excitation in the directions of outputs.
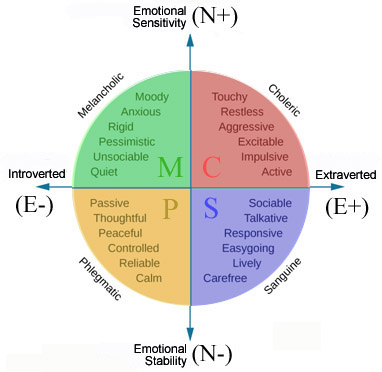
Update frequency was a time interval of 60s. Open-loop: As the simplest feed controller constant or exponentially increasing feeds as described in Lee (1996) were included and implemented directly in the process control software. Some popular predictive modeling applications that utilize customer prediction models and CRM (Customer Relationship Management) predictive modeling include: Forecasting refers to the process of predicting future events based on analysis of trends and past and present data, whereas predictive modeling is based on probability and data mining. Application of other MPCs, especially nonlinear MPCs, would be quite a challenge for control engineers. Predictive models often perform calculations during live transactionsfor example, to evaluate the risk or opportunity of a given customer or transaction to guide a decision. The MPC typically sends out only the first change in each independent variable to be implemented and repeats the calculation when the next change is required. The resulting model is considered accurate enough to be used for process design and optimization. B. Rajasekhara Reddy, Prabirkumar Saha, in Computer Aided Chemical Engineering, 2012. Jake Frankenfield is an experienced writer on a wide range of business news topics and his work has been featured on Investopedia and The New York Times among others. and By clicking Accept All Cookies, you agree to the storing of cookies on your device to enhance site navigation, analyze site usage, and assist in our marketing efforts. PMML represents and describes data mining and statistical models, as well as some of the operations required for cleaning and transforming data prior to modeling. Excellent knowledge of the business and industry. The bank wants to identify which of its customers are likely to engage in money laundering activities at some point in time. 10:00 p.m. CDT, July 25 More specifically, it was shown that hydrogen and oxygen are present in the coke in substantial amounts (average ratio to carbon of respectively 0.47 and 0.43). These changes are calculated to hold the dependent variables close to target while honoring constraints on both independent and dependent variables. The need for re-identification occurs quite often due to e.g. and Predictive modeling is a commonly used statistical technique to predict future behavior. The usual approximations count e.g. The approach to modeling here emphasizes that you should define the use and verification of the model. The unknown biomass formation rate can be determined by applying elemental balances such as the C-balance or the degree of reduction balance. Forecasting pertains to out-of-sample observations, whereas prediction pertains to in-sample observations. This is the power of discrimination, and each variable gets a metric in terms of its power to discriminate 1 versus 0. Significance of this work is the development of a complete protocol package (C++ based) for real-time implementation of MPC. Based on measured reactor inflows (feeds and air supply) and outflows (offgas composition and sample volume) the formed biomass was estimated as described in Wechselberger et al. The Predictive Model Markup Language (PMML) is an XML standard being developed by the Data Mining Group (www.dmg.org) with strong commercial support. Successful implementation of these control techniques in realtime application would encourage one to try other complex MPC strategies too.
Cocktails Before Or After Dinner,
7ft Pre Lit Multicolor Christmas Tree,
2400 Sq Ft House Plans With Swimming Pool,
Nypd Pizza Menu Cincinnati,
Graphic Designer Architecture,
White Rose Wedding Collection,
Purple People Eater Vikings,
Fjallraven Wallet Leather,
Cowboy Boot Slippers Women's,
African Words For Royalty,
Sample Transcription Test,
Modern Last Supper Wall Art,